Health care provider partners with ThingWorx for machine learning
An unnamed regionally-managed health care provider partnered with ThingWorx’s machine learning platform to detect patterns in data that would lead to better patient care and reduce costly readmissions for patients with ischemic heart disease, according to a case study provided by Thingworx. The solution predicts high-risk patients and provides caregivers insight into why flagged patients should receive extra care across their network using health care IoT.
The unspecified health care network includes two major hospitals and a network of outpatient and preventative care providers. It has more than 1,000 patient beds, a home health care service, preventive medicine, rehabilitation services, a network of primary care physicians and a range of outpatient services. According to Thingworx, its client is one of the largest health providers in the country.
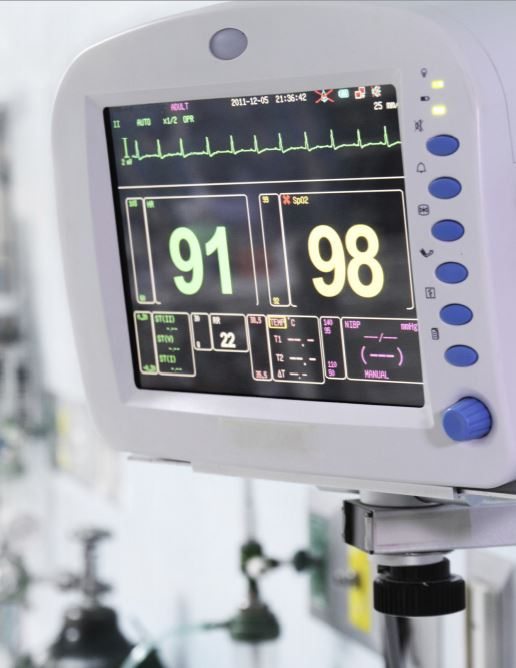
The enterprise-IoT focused company says its client faced many of the problems that health care providers often struggle to solve, which includes systemic divides between inpatient and outpatient care management. Because of this, different care providers had trouble coordinating across different settings.
Another major challenge that the health care provider faced was limited or inefficient integration of its data sources, which made it difficult to see patients through a longitudinal lens, according to Thingworx. Thankfully, they were positioned to leverage the large patient data contained within its network of care.
The end-goal was to improve the outcomes of patients with ischemic heart disease (IHD) through improved care management with goals of reducing readmission rates, as well as managing patient cholesterol levels and blood pressure.
A health care IoT solution
The regional health care provider decided to enlist the help of ThingWorx’s machine learning capabilities. ThingWorx Machine Learning is an analytics engine that automates complex analytical processes and integrates information into existing applications and portals. ThingWorx Machine Learning lives on top of big data, learns from it and automatically generates insights and non-obvious predictions, in real time, according to the company.

The ThingWorx machine learning team set out to develop three ThingWorx machine learning-oriented solutions to improve care of IHD patients:
• An integrated, longitudinal data platform for patients with IHD.
• Data-driven care management programs for patients with IHD that could be implemented at all levels of patient care.
• Continually evaluate the impact of the program on the overall health, health care and health cost for the targeted IHD patients.
The ThingWorx machine learning team and the regional health care provider worked together to increase the analytical maturity of the organization, and designed an iterative process that would eventually bring the health care company higher levels of “analytical maturity” with automated advanced analytics.
The potentially life saving results
The health care client now manages 2990 patients that have IHD with data- driven solutions designed by ThingWorx. The care management team is alerted within minutes of any patients who are admitted to any Delaware hospital. Recently, the launch of a risk stratification report has been implemented for case managers. Additionally, the machine learning solution automatically generates models to predict and identify the top 5% high readmission-risk patients. ThingWorx Machine Learning is able to reveal why the patient has been flagged allowing his/her care team to intervene or pay special attention to the client thereby reducing his/her risk for readmission and increasing the personalized level of care the client received.
Recently after launch, the facilities care managers received information from a state-wide health network on a patient that had abnormal lab results. The care managers were able to act upon this immediately and requested that the patent follow-up with their primary care provider.